※ GPSD INTRODUCTION:
Dynamic protein phosphorylation and dephosphorylation are essential regulatory mechanisms that ensure proper cellular signaling and biological processes. Protein dephosphorylation, catalyzed by phosphoprotein phosphatases (PPPs), is one of the most important post-translational modifications (PTMs), involved in regulation of signal transduction.
Here, we report a novel server, Group-based Prediction System for prediction of phosphatase-specific Dephosphorylation sites (GPSD), for prediction of PPP-specific dephosphorylation sites in eukaryotes. First, we pre-trained a general model using 561,416 non-redundant p-sites in 82,468 proteins. Then, transfer learning was conducted to obtain 103 PPP-specific predictors at the family, subfamily and single PPP levels, and 2 general predictors, using a well-curated data set of 4267 known site-specific phosphatase-substrate relations (ssPSRs) in 3304 dephosphorylation sites from 1765 proteins. Ten types of sequence features were extracted and integrated using two machine learning algorithms: penalized logistic regression (PLR) and deep neural networks (DNN), within a small-sample learning framework. To encode the contextual information of dephosphorylation sites, we further incorporated Bidirectional Encoder Representations from Transformers (BERT) and Generative Pre-trained Transformer (GPT) models into our GPSD framework. Using a newly collected data set of 126 ssPSRs in 159 dephosphorylation sites, we compared other existing tools with GPSD, which exhibited a much higher accuracy on a number of well-studied PPPs. For users, one or multiple protein sequences could be inputted in the FASTA format, and the output will be shown in a tabular list. Besides the basic statistics, we also integrated the knowledge from public resources to annotate the prediction results, including the experimental evidence, physical interactions, sequence logos, and dephosphorylation sites in sequences and 3D structures.
For the help of GPSD and the tutorial, please refer to the USER GUIDE page.
For the source code of GPSD, please visit the GitHub page.
▼ Example
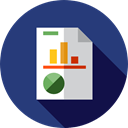